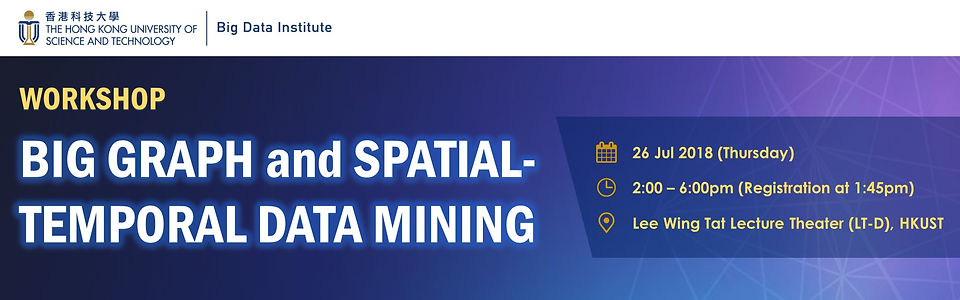
13:45-14:00
14:00-14:05
14:05-14:10
14:10-15:00
15:00-15:50
15:50-16:40
16:40-17:00
AGENDA
Registration
Welcome Remarks
Prof Lei Chen, Acting Director, HKUST Big Data Institute &
Professor, Department of Computer Science and Engineering, HKUST
Opening Remarks
Prof DY Yeung, Acting Head & Professor,
Department of Computer Science and Engineering, HKUST
Unintended Consequences of Disclosing Location Data
Prof Cyrus Shahabi, Professor of Computer Science, Electrical Engineering and Spatial Sciences & Chair,
Department of Computer Science, University of Southern California
Towards Big Graph Processing: Applications, Challenges, and Advances
Prof Xuemin Lin, Scientia Professor, FIEEE, Database Research Group,
School of Computer Science and Engineering, University of New South Wales
Persistent Community Search in Temporal Networks
Prof Jeffrey Xu Yu, Professor,
Department of Systems Engineering and Engineering Management,
The Chinese University of Hong Kong
17:00-18:00
18:00
Coffee Break
Mining Structured Knowledge from Massive Text Data: A Weakly Supervised Approach
Prof Jiawei Han, Abel Bliss Professor,
Department of Computer Science, University of Illinois at Urbana-Champaign
End of Program
* The event program is subject to change without prior notice
SPEAKERs & Abstracts
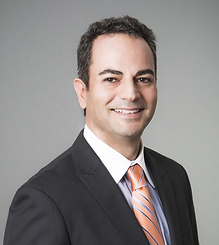
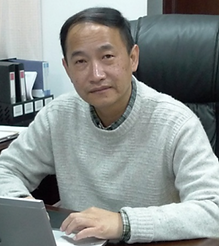

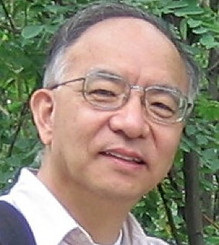
Prof Cyrus Shahabi
Professor of Computer Science, Electrical Engineering and Spatial Sciences & Chair, Department of Computer Science, University of Southern California
Cyrus Shahabi is a Professor of Computer Science, Electrical Engineering and Spatial Sciences, and the chair of the Computer Science Department. He is also the Director of the Integrated Media Systems Center (IMSC) and the Informatics Program at USC’s Viterbi School of Engineering. He was the CTO and co-founder of a USC spin-off, Geosemble Technologies, which was acquired in July 2012. Since then, he founded another company, ClearPath (recently rebranded as TallyGo), focusing on predictive path-planning for car navigation systems. He received his B.S. in Computer Engineering from Sharif University of Technology in 1989 and then his M.S. and Ph.D. Degrees in Computer Science from the University of Southern California in May 1993 and August 1996, respectively. He authored two books and more than three hundred research papers in databases, GIS and multimedia with more than 12 US Patents.
Dr. Shahabi was an Associate Editor of IEEE Transactions on Parallel and Distributed Systems (TPDS) from 2004 to 2009, IEEE Transactions on Knowledge and Data Engineering (TKDE) from 2010-2013 and VLDB Journal from 2009-2015. He is currently the chair of ACM SIGSPATIAL for the 2017-2020 term and also on the editorial board of the ACM Transactions on Spatial Algorithms and Systems (TSAS) and ACM Computers in Entertainment. He is the founding chair of IEEE NetDB workshop and also the general co-chair of SSTD’15, ACM GIS 2007, 2008 and 2009. He chaired the nomination committee of ACM SIGSPATIAL for the 2011-2014 terms. In the past, he has been PC co-chair of several conferences such as APWeb+WAIM’2017, BigComp’2016, MDM’2016, DASFAA 2015, IEEE MDM 2013 and IEEE BigData 2013, and regularly serves on the program committee of major conferences such as VLDB, SIGMOD, IEEE ICDE, ACM SIGKDD, IEEE ICDM, and ACM Multimedia.
Dr. Shahabi is a fellow of IEEE, and a recipient of the ACM Distinguished Scientist award in 2009, the 2003 U.S. Presidential Early Career Awards for Scientists and Engineers (PECASE), the NSF CAREER award in 2002, and the 2001 Okawa Foundation Research Grant for Information and Telecommunications. He was also a recipient of the US Vietnam Education Foundation (VEF) faculty fellowship award in 2011 and 2012, an organizer of the 2011 National Academy of Engineering “Japan-America Frontiers of Engineering” program, an invited speaker in the 2010 National Research Council (of the National Academies) Committee on New Research Directions for the National Geospatial-Intelligence Agency, and a participant in the 2005 National Academy of Engineering “Frontiers of Engineering” program.
Talk abstract:This talk consists of two parts. In the first part, we show it is possible to infer social behaviors by observing people’s behaviors in the real world. In particular, we focus on inferring pair-wise strength as the strength of social connections between a pair of users by utilizing the available high-fidelity location data representing people’s movements. Towards this end, we utilize the information generated by simultaneous occurrences of individuals in the same geographic locale at roughly the same time, termed co-locations. Subsequently, we argue that the users may be willing to share location data to benefit from Location-Based-Services but unwilling to reveal their social connections. Therefore, in the second part of the talk, we aim to hide user co-locations, without sacrificing the quality and effectiveness of services that finance the location-based applications. To this end, we propose three different mechanisms that create confusion in the information of a user’s co-locating partner through obfuscation of his spatial and temporal positions. The inference attack takes into account the prior information that might be available to an adversary along with the information leaked by the obfuscation mechanism, thereby enabling an accurate and efficient assessment of the co-location privacy risk stemming from the use of location-based services.
Prof Xuemin Lin
Scientia Professor, FIEEE, Database Research Group,
School of Computer Science and Engineering, University of New South Wales
Xuemin Lin is a UNSW Scientia Professor, the head of database group in the school of computer science and engineering at UNSW, and a current Professor at ECNU (specially appointed by the Chinese National Thousands Distinguished Professors Program). He is a fellow of IEEE. Xuemin's research interests lie in databases, data mining, algorithms, and complexities. Specifically, he is working in the areas of scalable processing and mining of various data, including graph, spatial-temporal, streaming, text and uncertain data.
Xuemin has been very frequently serving as a PC member and area chairs (senior PC members) in SIGMOD, VLDB, ICDE, ICDM, KDD, CIKM, and EDBT. He received the honour of outstanding reviewer in KDD2012. He was an associate editor of ACM TODS (2008-2014) and IEEE TKDE (Feb 2013- Jan 2015), and an associate editor-in-Chief of TKDE (2015-2016), respectively. Currently, he is the editor-in-Chief of TKDE (Jan 2017 - now) and an associate editor of WWW Journal (2013 - now).
Talk abstract: Graph data are key parts of Big Data and widely used for modelling complex structured data with a broad spectrum of applications. Over the last decade, tremendous research efforts have been devoted to many fundamental problems in managing and analysing graph data. In this talk, I will cover various applications, challenges, and recent advances. We will also look to the future of the area.
Prof Jeffrey Xu Yu
Professor,
Department of Systems Engineering and Engineering Management,
The Chinese University of Hong Kong
Dr. Jeffrey Xu Yu is a Professor in the Department of Systems Engineering and Engineering Management, The Chinese University of Hong Kong. His current main research interests include graph mining, graph query processing, graph pattern matching, keywords search in databases, and online social networks. Dr. Yu served as an Information Director and a member in ACM SIGMOD executive committee (2007-2011), an associate editor of IEEE Transactions on Knowledge and Data Engineering (2004-2008), and an associate editor in VLDB Journal (2007-2013). Currently he serves as an associate editor of ACM Transactions on Database Systems (TODS), WWW Journal, Data Science and Engineering, the International Journal of Cooperative Information Systems, the Journal on Health Information Science and Systems (HISS), and Journal of Information Processing. Dr. Yu served/serves in many organization committees and program committees in international conferences/workshops including PC Co-chair of APWeb'04, WAIM'06, APWeb/WAIM'07, WISE'09, PAKDD'10, DASFAA'11, ICDM'12, NDBC'13, ADMA'14, CIKM'15 and Bigcomp17, and conference general Co-chair of APWeb'13 and ICDM’18.
Talk abstract: Community search is a fundamental graph mining task. In applications such as analysis of communication networks, collaboration networks, and social networks, edges are typically associated with timestamps. In this talk, we discuss the problem of findingpersistent communities in a temporal network, in which every edge is associated with a timestamp. The goal is to identify the communities that are persistent over time. To this end, we propose a new persistent community model to capture the persistence of a community. We will discuss the model, the hardness of the problem, and our solutions. We will also discuss some research issues related to temporal graphs in this talk.
Prof Jiawei Han
Abel Bliss Professor,
Department of Computer Science, University of Illinois at Urbana-Champaign
Jiawei Han is Abel Bliss Professor in the Department of Computer Science, University of Illinois at Urbana-Champaign. He has been researching into data mining, information network analysis, database systems, and data warehousing, with over 900 journal and conference publications. He has chaired or served on many program committees of international conferences in most data mining and database conferences. He also served as the founding Editor-In-Chief of ACM Transactions on Knowledge Discovery from Data and the Director of Information Network Academic Research Center supported by U.S. Army Research Lab (2009-2016), and is the co-Director of KnowEnG, an NIH funded Center of Excellence in Big Data Computing since 2014. He is Fellow of ACM, Fellow of IEEE, and received 2004 ACM SIGKDD Innovations Award, 2005 IEEE Computer Society Technical Achievement Award, and 2009 M. Wallace McDowell Award from IEEE Computer Society. His co-authored book "Data Mining: Concepts and Techniques" has been adopted as a textbook popularly worldwide.
Talk abstract: The real-world big data are largely unstructured, interconnected, and dynamic, in the form of natural language text. It is highly desirable to transform such massive unstructured data into structured knowledge. Many researchers rely on labor-intensive labeling and curation to extract knowledge from such data. However, such approaches may not be scalable, especially considering that a lot of text corpora are highly dynamic and domain-specific. We argue that massive text data itself may disclose a large body of hidden patterns, structures, and knowledge. Equipped with domain-independent and domain-dependent knowledge-bases, we should explore the power of massive data itself for turning unstructured data into structured knowledge.
We introduce a set of methods developed recently in our own group on exploration the power of big text data, including mining quality phrases, recognition and typing of entities and relations by weak supervision, pattern-based entity-attribute-value extraction, multi-faceted taxonomy discovery, and construction of multi-dimensional text cubes. We show the massive text data itself can be powerful at disclosing patterns and structures, and it is promising to explore the power of massive text data to turn massive text data to structured knowledge.
Map
Jul 26, 2:00 PM – 6:00 PM |
Lee Wing Tat Lecture Theater (LT-D), HKUST
Contact Us
To contact us for this event, please email to bdi@ust.hk or contact us at (852) 2358 8334.